The Evolution of Business Intelligence: From Basic Reporting to Predictive Analytics
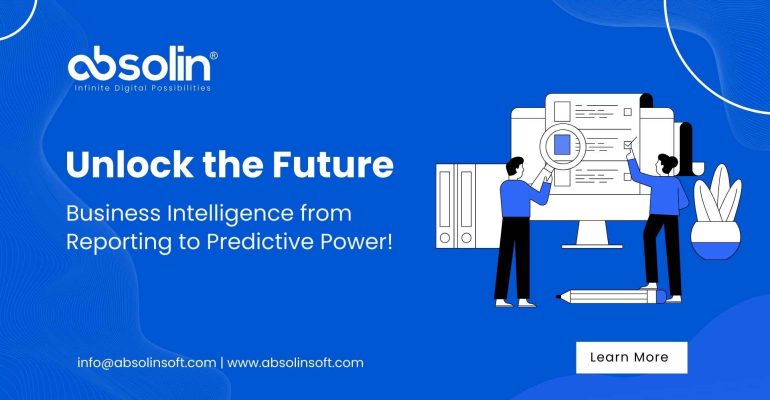
The Evolution of Business Intelligence: From Basic Reporting to Predictive Analytics
Business Intelligence (BI) has transformed significantly over the past few decades. What began as a tool for basic reporting has now evolved into a comprehensive system that incorporates predictive analytics and artificial intelligence (AI) to guide businesses in making informed decisions. This evolution mirrors the growing complexity of data, advancements in technology, and the rising demands for actionable insights. Here’s a look at how BI has progressed and the major milestones in its development:
1. Basic Reporting: The Origins of BI
In the early stages, business intelligence was largely focused on static reporting. Organizations relied on simple tools to generate reports from their data repositories, helping them review past performance. This kind of BI was primarily descriptive, summarizing what had happened based on historical data.
Key Features:
- Manual data collection and aggregation.
- Limited to spreadsheets and basic databases.
- Focused on creating reports and dashboards to review business metrics.
- Reports were generated periodically, such as daily, weekly, or monthly.
While these reports helped businesses monitor their operations, they were limited in scope and lacked real-time insights.
2. Dynamic Dashboards and OLAP: The Next Step
As businesses accumulated more data, the need for more sophisticated tools emerged. Enter Online Analytical Processing (OLAP), a technology that allowed for faster, multidimensional analysis of large data sets. This development enabled users to view and manipulate data in real time, drilling down into specific metrics.
Key Features:
- Real-time data querying and interactive dashboards.
- OLAP cubes allowed data to be sliced and diced across multiple dimensions.
- Visualization tools that made data more accessible for non-technical users.
The introduction of dashboards provided decision-makers with a bird’s-eye view of key metrics, enabling them to track performance without waiting for periodic reports.
3. Self-Service BI: Empowering Non-Technical Users
As BI tools became more advanced, there was a growing demand for solutions that could be used by non-technical employees. This marked the rise of self-service BI tools, such as Tableau and Power BI, which allowed users to generate their own reports and perform data analysis without needing extensive coding knowledge.
Key Features:
- Drag-and-drop interfaces for easier data manipulation.
- Customizable reports and dashboards.
- Integration with a wide range of data sources.
- Users could analyze data without needing IT teams to pull reports.
Self-service BI democratized data analytics, putting powerful tools in the hands of business managers, sales teams, and marketers.
4. Advanced Analytics and Data Mining
As businesses recognized the value of data, they began looking beyond historical analysis toward predictive and prescriptive analytics. This stage involved the application of data mining techniques, which allowed businesses to identify hidden patterns and trends within their data.
Key Features:
- Predictive models that forecast future trends.
- Use of algorithms to extract insights from large data sets.
- Prescriptive analytics that suggested actions based on data.
- Integration of machine learning (ML) to automate insights and recommendations.
By using advanced analytics, businesses could not only understand what had happened in the past but also predict what was likely to happen in the future, enabling proactive decision-making.
5. Predictive Analytics: The Modern Era of BI
Today, BI is defined by its ability to provide predictive analytics. This is where BI moves from being descriptive (explaining what happened) to predictive (anticipating future outcomes) and even prescriptive (recommending actions). Modern BI systems use AI and machine learning to analyze past data and forecast trends, consumer behaviors, and potential challenges.
Key Features:
- Integration of AI and machine learning algorithms.
- Predictive analytics that forecast sales, market trends, and operational issues.
- Prescriptive analytics that provide actionable insights.
- Real-time data analysis for instant decision-making.
With predictive analytics, businesses can automate decision-making processes, identify growth opportunities, and mitigate risks with data-driven foresight.
6. The Future: AI-Powered BI and Beyond
As BI continues to evolve, the next frontier lies in AI-powered BI and augmented analytics. These advancements will focus on automating the entire data lifecycle—from collection and cleaning to analysis and visualization. AI will allow businesses to make even more accurate predictions and decisions, with systems capable of learning and improving over time.
Key Features:
- Natural language processing (NLP) to allow users to query data using plain language.
- AI-driven insights that require no human intervention.
- Automated data storytelling to present insights in a narrative form.
- Continuous learning models that refine predictions based on new data.
Conclusion
The evolution of Business Intelligence from basic reporting to advanced predictive analytics has revolutionized the way businesses operate. By embracing the latest BI technologies, organizations can not only look back at what has happened but also anticipate future challenges and opportunities, empowering them to make smarter, data-driven decisions. As BI continues to evolve, businesses that invest in these tools will be better positioned to thrive in an increasingly data-centric world.